Data-Driven Strategies for Enhancing Customer Retention in E-commerce
DOI:
https://doi.org/10.62802/qjp26v22Keywords:
Customer retention, e-commerce, data-driven strategies, predictive analytics, machine learning, personalization, customer engagement, loyalty programs, churn reduction, customer lifetime value (LTV)Abstract
In the highly competitive e-commerce landscape, customer retention is critical for sustaining business growth and profitability. This research explores data-driven strategies for enhancing customer retention by leveraging advanced analytics, machine learning models, and behavioral data. By understanding customer preferences, purchase patterns, and engagement behaviors, businesses can implement personalized marketing campaigns, loyalty programs, and product recommendations. The study examines how predictive analytics can identify at-risk customers and proactively address their needs through targeted interventions. Additionally, it highlights the role of real-time data processing and customer feedback analysis in optimizing retention strategies. Challenges such as data privacy concerns, algorithmic bias, and the ethical use of customer data are also addressed. This research aims to provide actionable insights for e-commerce businesses, enabling them to foster long-term customer relationships, reduce churn rates, and improve lifetime value (LTV) metrics. By combining technological innovation with customer-centric practices, the study offers a comprehensive framework for creating meaningful and sustained customer engagement in the digital age.
References
Amosu, O. R., Kumar, P., Fadina, A., Ogunsuji, Y. M., Oni, S., Faworaja, O., & Adetula, K. (2024). Data-driven personalized marketing: Deep Learning in Retail and E-commerce. World Journal of Advanced Research and Reviews, 23(02), 788-796.
Boppana, V. R. (2021). Innovative CRM Strategies for Customer Retention in E-Commerce. ESP Journal of Engineering & Technology Advancements (ESP-JETA), 1(1), 173-183.
Gayam, S. R. (2022). Enhancing Customer Retention in E-Commerce with AI: Techniques for Predictive Analytics, Personalization, and Loyalty Programs. Australian Journal of Machine Learning Research & Applications, 2(2), 303-342.
Gonzalez, M., & Rabbi, F. (2023). Evaluating the impact of Big Data Analytics on personalized E-commerce shopping experiences and customer retention strategies. Journal of Computational Social Dynamics, 8(2), 13-25.
Nalla, L. N., & Reddy, V. M. (2024). AI-Driven Big Data Analytics for Enhanced Customer Journeys: A New Paradigm in E-Commerce. International Journal of Advanced Engineering Technologies and Innovations, 1(2), 719-740.
Reddy, V. M., & Nalla, L. N. (2024). Personalization in E-Commerce Marketing: Leveraging Big Data for Tailored Consumer Engagement. Revista de Inteligencia Artificial en Medicina, 15(1), 691-725.
Saleem, H., Uddin, M. K. S., Habib-ur-Rehman, S., Saleem, S., & Aslam, A. M. (2019). Strategic data driven approach to improve conversion rates and sales performance of e-commerce websites. International Journal of Scientific & Engineering Research, 10(4), 588-593.
Saini, K., & Singh, A. (2024, June). Data-Driven Strategies for Improving Customer Engagement and Retention in E-commerce. In 2024 First International Conference on Technological Innovations and Advance Computing (TIACOMP) (pp. 499-506). IEEE.
Shobana, J., Gangadhar, C., Arora, R. K., Renjith, P. N., Bamini, J., & devidas Chincholkar, Y. (2023). E-commerce customer churn prevention using machine learning-based business intelligence strategy. Measurement: Sensors, 27, 100728.
Tran, M. T. (2024). Unlocking the AI-Powered Customer Experience: Personalized Service, Enhanced Engagement, and Data-Driven Strategies for E-Commerce Applications. In Enhancing and Predicting Digital Consumer Behavior with AI (pp. 375-382). IGI Global.
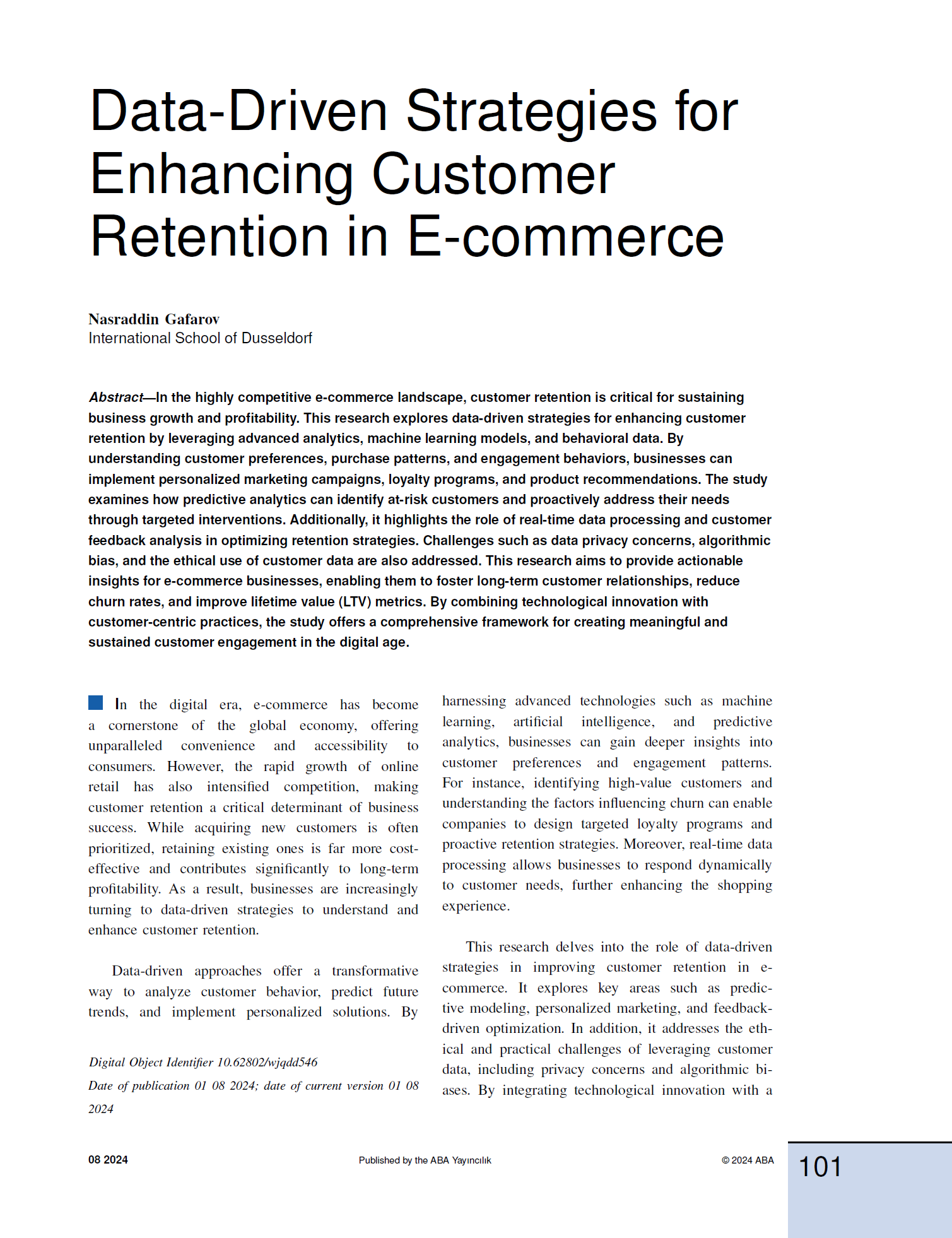