Business Analytics Models in the Tech Corporates from Automative Sectors to Semiconductor Sectors
DOI:
https://doi.org/10.62802/hadkw970Keywords:
business analytics, automotive industry, semiconductor industry, predictive modeling, supply chain optimization, Industry 4.0, AI-driven manufacturing, data-driven strategies, operational agilityAbstract
The integration of business analytics models in technology-driven corporations across automotive and semiconductor sectors is redefining decision-making, enhancing operational efficiency, and driving innovation. These models leverage advanced analytics techniques, including predictive modeling, machine learning, and optimization algorithms, to process complex datasets and generate actionable insights. In the automotive sector, analytics models facilitate advancements in supply chain optimization, predictive maintenance, and autonomous vehicle development. Similarly, in the semiconductor industry, analytics plays a pivotal role in yield optimization, defect detection, and demand forecasting. This research examines the diverse applications of business analytics across these sectors, highlighting how data-driven strategies address industry-specific challenges such as volatile demand, technological complexities, and competitive pressures. It also explores the interplay between analytics and emerging trends, such as Industry 4.0 and AI-driven manufacturing. By evaluating case studies and frameworks, the study provides insights into the strategic adoption of analytics to enhance decision-making and operational agility. The findings emphasize the transformative potential of business analytics in tech-driven industries, paving the way for smarter, more resilient corporate strategies.
References
Al Tareq, A., Rana, M. J., Mostofa, M. R., & Rahman, M. S. (2024). Impact of IoT and Embedded System on Semiconductor Industry A Case Study. Control Systems and Optimization Letters, 2(2), 211-216.
Garcia, J. A. C., Arvidsson, A., & Jonsson, P. (2024). An analysis of automakers navigating an evolving semiconductor landscape. International Journal of Physical Distribution & Logistics Management, 54(6), 586-609.
Harb, A. (2024). Creating an Effective Digital Supply Chain Strategy for the Technology Industry in the Era of Industry 4.0 (Doctoral dissertation, Golden Gate University).
Kurrahman, T., Tsai, F. M., Jeng, S. Y., Chiu, A. S., Wu, K. J., & Tseng, M. L. (2024). Sustainable development performance in the semiconductor industry: A data-driven practical guide to strategic roadmapping. Journal of Cleaner Production, 445, 141207.
Ni, H. (2024). Comparative Analysis of Three Semiconductor Technology Companies. Highlights in Business, Economics and Management, 24, 891-895.
Oliver, S. (2024). Transformative Dynamics: Industry 4.0 and IIoT's Comprehensive Impact on the Semiconductor Industry and Qualcomm's Snapdragon Evolution. Available at SSRN 4803683.
Ozturk, B. (2024). Time Series Models for Revenue Forecasting in the Semiconductor Industry: A Case Study of ASML.
Rath, K. C., Mishra, D., Tripathy, S. K. T., Mishra, B. K., & Muduli, K. (2025). Potential of AI, Quantum Computing, and Semiconductor Technology Adoption in Future Industries: Scope, Challenges, and Opportunities. Integration of AI, Quantum Computing, and Semiconductor Technology, 415-440.
Rogers, A., Dibsie, C., Kuzneski, E., Underwood, D., Brozey, R., Sullivan, L., ... & Porter, M. D. (2024, May). Forecasting Breakthroughs: Identifying Future Leaders in the Semiconductor Industry. In 2024 Systems and Information Engineering Design Symposium (SIEDS) (pp. 425-430). IEEE.
Ryu, S., Kwak, K., & Lee, S. (2024). The growth patterns of firms in the industrial value chain using the hidden markov model: Evidence from the Korean semiconductor industry. Computers & Industrial Engineering, 192, 110187.
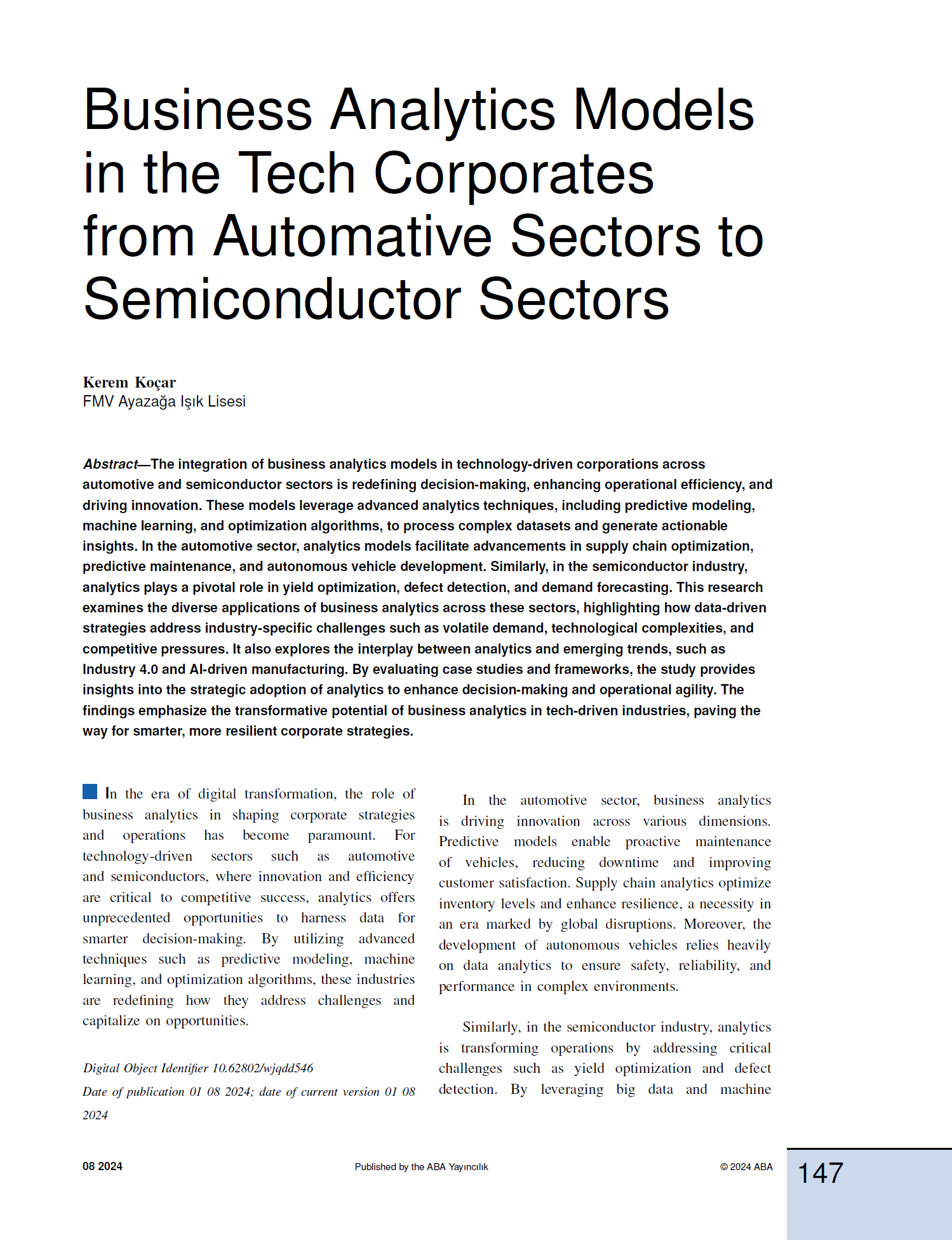