AI in Biomedical Imaging and Diagnostics
DOI:
https://doi.org/10.62802/fene2356Keywords:
Artificial intelligence, biomedical imaging, diagnostics, synthetic biology, T-cell engineering, immunotherapy, tumor microenvironment, antigen recognition, disease biomarkers, personalized medicineAbstract
Advances in artificial intelligence (AI) and synthetic biology have profoundly influenced biomedical research, creating transformative opportunities in imaging, diagnostics, and therapeutic engineering. In biomedical imaging, AI-driven algorithms enhance precision and accuracy, enabling automated analysis of complex datasets, real-time imaging insights, and identification of disease biomarkers. Meanwhile, synthetic biology redefines cellular engineering, particularly in T-cell research, by enabling customized functionalities, such as precision-targeted antigen recognition and tunable immune responses. The integration of AI into T-cell engineering amplifies these capabilities, facilitating the design and optimization of synthetic circuits, predictive modeling of cellular behaviors, and dynamic monitoring of therapeutic outcomes. This interdisciplinary approach is revolutionizing diagnostics and immunotherapy by streamlining the identification of disease-specific markers, improving diagnostic accuracy, and enabling real-time modulation of T-cell functionality within the tumor microenvironment. By combining AI-powered insights with synthetic biology's ability to engineer living systems, this research aims to address critical challenges in disease treatment, including tumor heterogeneity and immune evasion. This work explores the synergistic application of AI and synthetic biology in biomedical imaging and T-cell engineering, highlighting state-of-the-art technologies, their therapeutic potential, and the future landscape of personalized medicine.
References
Alkayyali, Z. K., Taha, A. M., Zarandah, Q. M., Abunasser, B. S., Barhoom, A. M., & Abu-Naser, S. S. (2024). Advancements in AI for Medical Imaging: Transforming Diagnosis and Treatment.
Bai, A., Si, M., Xue, P., Qu, Y., & Jiang, Y. (2024). Artificial intelligence performance in detecting lymphoma from medical imaging: a systematic review and meta-analysis. BMC Medical Informatics and Decision Making, 24(1), 13.
Dodda, S., Chintala, S., Kanungo, S., Adedoja, T., & Sharma, S. (2024). Exploring AI-driven Innovations in Image Communication Systems for Enhanced Medical Imaging Applications. Journal of Electrical Systems, 20(3s), 949-959.
Hua, D., Petrina, N., Young, N., Cho, J. G., & Poon, S. K. (2024). Understanding the factors influencing acceptability of AI in medical imaging domains among healthcare professionals: A scoping review. Artificial Intelligence in Medicine, 147, 102698.
Kasula, B. Y. (2024). Advancements in AI-driven Healthcare: A Comprehensive Review of Diagnostics, Treatment, and Patient Care Integration. International Journal of Machine Learning for Sustainable Development, 6(1), 1-5.
Obuchowicz, R., Strzelecki, M., & Piórkowski, A. (2024). Clinical Applications of Artificial Intelligence in Medical Imaging and Image Processing—A Review. Cancers, 16(10), 1870.
Prabhod, K. J., & Gadhiraju, A. (2024). Foundation Models in Medical Imaging: Revolutionizing Diagnostic Accuracy and Efficiency. Journal of Artificial Intelligence Research and Applications, 4(1), 471-511.
Sharma, A. K., Chanderwal, N., Tyagi, S., & Upadhyay, P. (Eds.). (2024). Future of AI in Medical Imaging. IGI Global.
Tayebi Arasteh, S., Ziller, A., Kuhl, C., Makowski, M., Nebelung, S., Braren, R., ... & Kaissis, G. (2024). Preserving fairness and diagnostic accuracy in private large-scale AI models for medical imaging. Communications Medicine, 4(1), 46.
Zhang, Y., Feng, H., Zhao, Y., & Zhang, S. (2024). Exploring the application of the artificial-intelligence-integrated platform 3D Slicer in medical imaging education. Diagnostics, 14(2), 146.
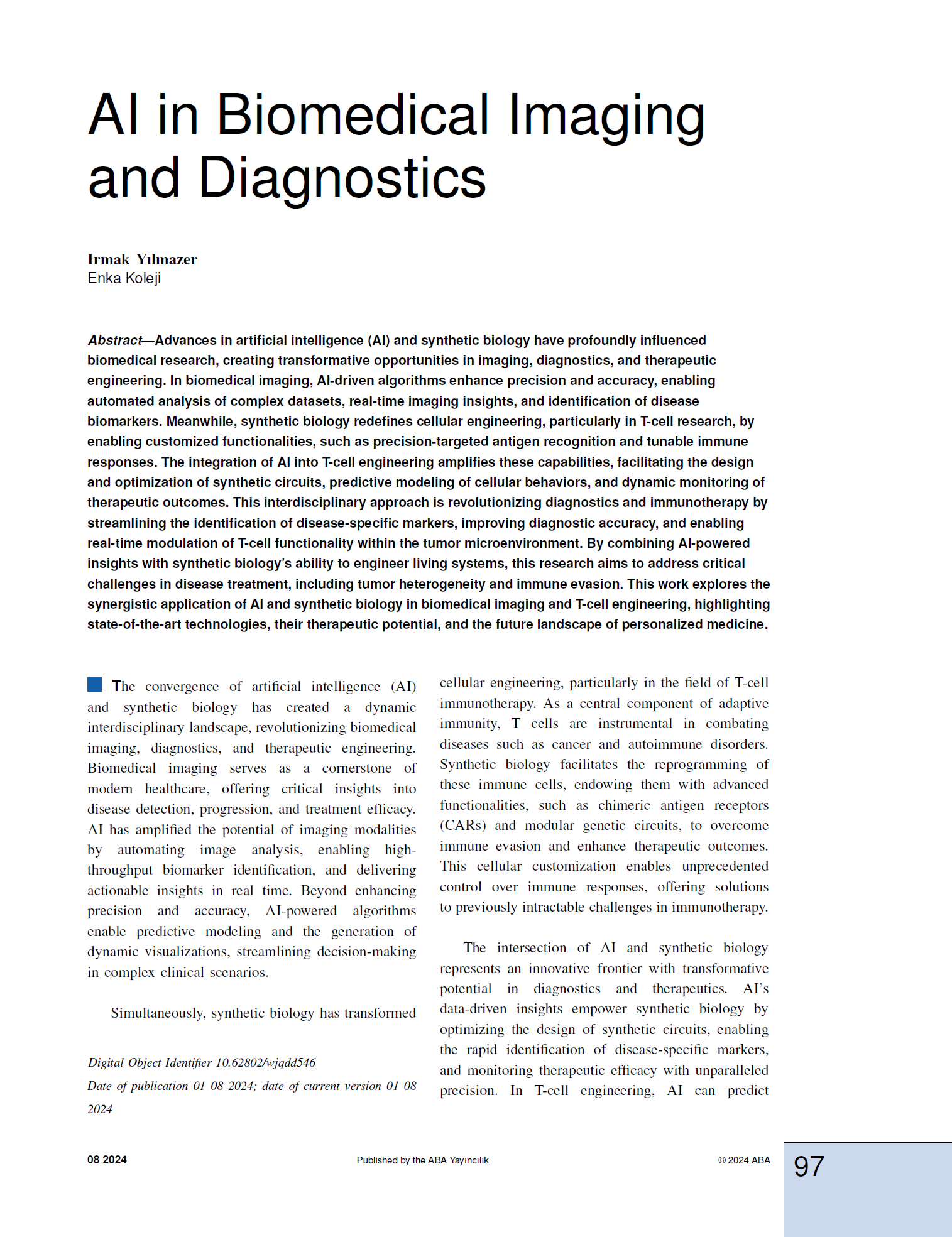