Predictive Analytics for Sales Forecasting and Inventory Management
DOI:
https://doi.org/10.62802/7t6wq430Keywords:
Predictive analytics, sales forecasting, inventory management, demand forecasting, machine learning, time series analysis, operational efficiency, data-driven decision-making, profitability, real-time analysisAbstract
Predictive analytics has become a cornerstone of modern business operations, particularly in the domains of sales forecasting and inventory management. By leveraging historical data, machine learning algorithms, and statistical models, predictive analytics empowers businesses to anticipate future demand, optimize inventory levels, and enhance operational efficiency. This research explores the integration of predictive analytics into sales and inventory processes, focusing on its ability to reduce waste, minimize stockouts, and improve profitability. Key areas of investigation include the application of time series analysis, regression models, and AI-driven demand forecasting. The study also examines challenges such as data quality, integration complexities, and the need for real-time analysis in dynamic market conditions. Through case studies and industry insights, this research highlights best practices for implementing predictive analytics to achieve data-driven decision-making. Ultimately, it provides a roadmap for businesses to align predictive capabilities with strategic objectives, creating a more agile and competitive operational framework.
References
Akın, M. (2024). AI-Based Inventory Management Solutions for American Manufacturing and Retail: Techniques and Real-World Applications. Distributed Learning and Broad Applications in Scientific Research, 10, 132-148.
Gupta, A., & Agarwal, P. (2024, May). Enhancing sales forecasting accuracy through integrated enterprise resource planning and customer relationship management using artificial intelligence. In 2024 3rd International Conference on Artificial Intelligence For Internet of Things (AIIoT) (pp. 1-6). IEEE.
Hasan, M. R. (2024). Addressing Seasonality and Trend Detection in Predictive Sales Forecasting: A Machine Learning Perspective. Journal of Business and Management Studies, 6(2), 100-109.
Jack, F., & Bommu, R. (2024). Unveiling the Potential: AI-Powered Dynamic Inventory Management in the USA. International Journal of Advanced Engineering Technologies and Innovations, 1(3), 241-261.
Joel, O. T., & Oguanobi, V. U. (2024). Data-driven strategies for business expansion: Utilizing predictive analytics for enhanced profitability and opportunity identification. International Journal of Frontiers in Engineering and Technology Research, 6(02), 071-081.
Khan, A., Jhanjhi, N. Z., Haji, D. H. T. B. A., & Omar, H. A. H. B. H. (2024). Internet of Things (IoT) Impact on Inventory Management: A Review. Cybersecurity Measures for Logistics Industry Framework, 224-247.
Mittal, S. (2024). Framework for Optimized Sales and Inventory Control: A Comprehensive Approach for Intelligent Order Management Application. International Journal of Computer Trends and Technology, 72(3), 61-65.
Olaoye, G. (2024). Developing Predictive Models for Inventory Optimization (No. 13396). EasyChair.
Pasupuleti, V., Thuraka, B., Kodete, C. S., & Malisetty, S. (2024). Enhancing supply chain agility and sustainability through machine learning: Optimization techniques for logistics and inventory management. Logistics, 8(3), 73.
Reddy, V. M., & Nalla, L. N. (2024). Optimizing E-Commerce Supply Chains Through Predictive Big Data Analytics: A Path to Agility and Efficiency. International Journal of Machine Learning Research in Cybersecurity and Artificial Intelligence, 15(1), 555-585.
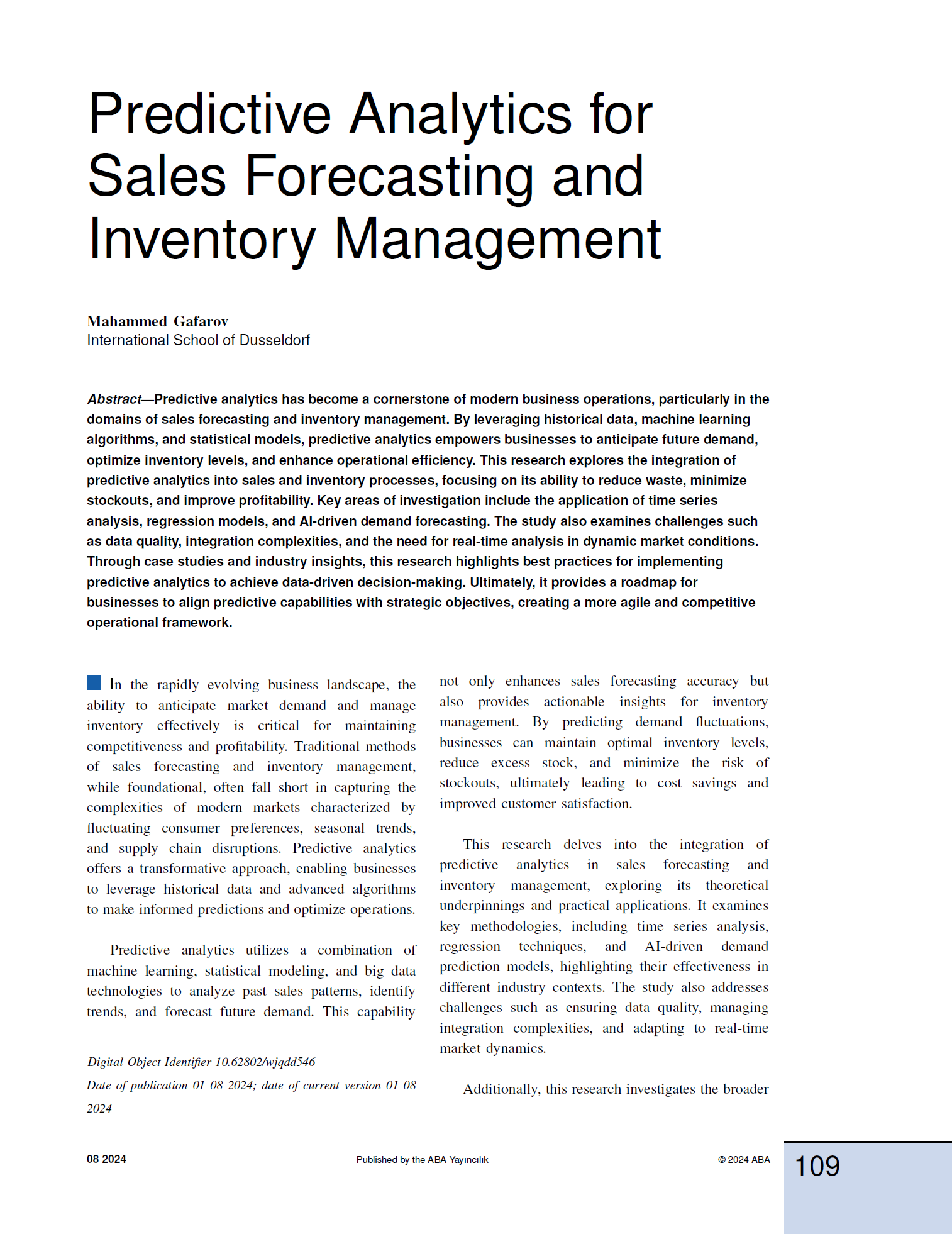