Machine Learning Integration in Econometric Models
DOI:
https://doi.org/10.62802/8c33p210Keywords:
Machine learning, econometrics, causal inference, predictive modeling, hybrid frameworks, regularization, deep learning, macroeconomic forecasting, policy evaluation, data analysisAbstract
The integration of machine learning (ML) into econometric models represents a transformative advancement in the field of econometrics, enabling researchers to tackle complex, high-dimensional datasets while maintaining the interpretability and rigor of traditional econometric approaches. This research investigates the synergies between machine learning and econometrics, focusing on how ML techniques can enhance model flexibility, predictive accuracy, and causal inference in economic analysis. By leveraging methods such as regularization, ensemble learning, and deep learning, the study explores applications in macroeconomic forecasting, policy evaluation, and market analysis. Furthermore, it addresses the challenges of balancing interpretability with predictive performance, emphasizing the need for hybrid frameworks that merge machine learning's adaptability with econometrics' theoretical foundation. The findings demonstrate the potential of ML-enhanced econometric models to revolutionize economic research and policy-making by providing robust, data-driven insights.
References
Challoumis, C. (2024, October). THE ECONOMICS OF AI-HOW MACHINE LEARNING IS DRIVING VALUE CREATION. In XVI International Scientific Conference (pp. 94-125).
Elomari, Y., Mateu, C., Marín-Genescà, M., & Boer, D. (2024). A data-driven framework for designing a renewable energy community based on the integration of machine learning model with life cycle assessment and life cycle cost parameters. Applied Energy, 358, 122619.
Li, Z., Wang, B., & Chen, Y. (2024). Incorporating economic indicators and market sentiment effect into US Treasury bond yield prediction with machine learning. Journal of Infrastructure, Policy and Development, 8(9), 7671.
Masoud, N. (2024). Artificial intelligence and unemployment dynamics: an econometric analysis in high-income economies. Technological Sustainability.
Omidkar, A., Alagumalai, A., Li, Z., & Song, H. (2024). Machine learning assisted techno-economic and life cycle assessment of organic solid waste upgrading under natural gas. Applied Energy, 355, 122321.
Shboul, B., Zayed, M. E., Ashraf, W. M., Usman, M., Roy, D., Irshad, K., & Rehman, S. (2024). Energy and economic analysis of building integrated photovoltaic thermal system: Seasonal dynamic modeling assisted with machine learning-aided method and multi-objective genetic optimization. Alexandria Engineering Journal, 94, 131-148.
Wang, N., Guo, Z., Shang, D., & Li, K. (2024). Carbon trading price forecasting in digitalization social change era using an explainable machine learning approach: The case of China as emerging country evidence. Technological Forecasting and Social Change, 200, 123178.
Xu, H., Li, S., Niu, K., & Ping, G. (2024). Utilizing deep learning to detect fraud in financial transactions and tax reporting. Journal of Economic Theory and Business Management, 1(4), 61-71.
Zhang, Z., Vo, D. N., Nguyen, T. B., Sun, J., & Lee, C. H. (2024). Advanced process integration and machine learning-based optimization to enhance techno-economic-environmental performance of CO2 capture and conversion to methanol. Energy, 293, 130758.
Zheng, H., Wu, J., Song, R., Guo, L., & Xu, Z. (2024). Predicting financial enterprise stocks and economic data trends using machine learning time series analysis.
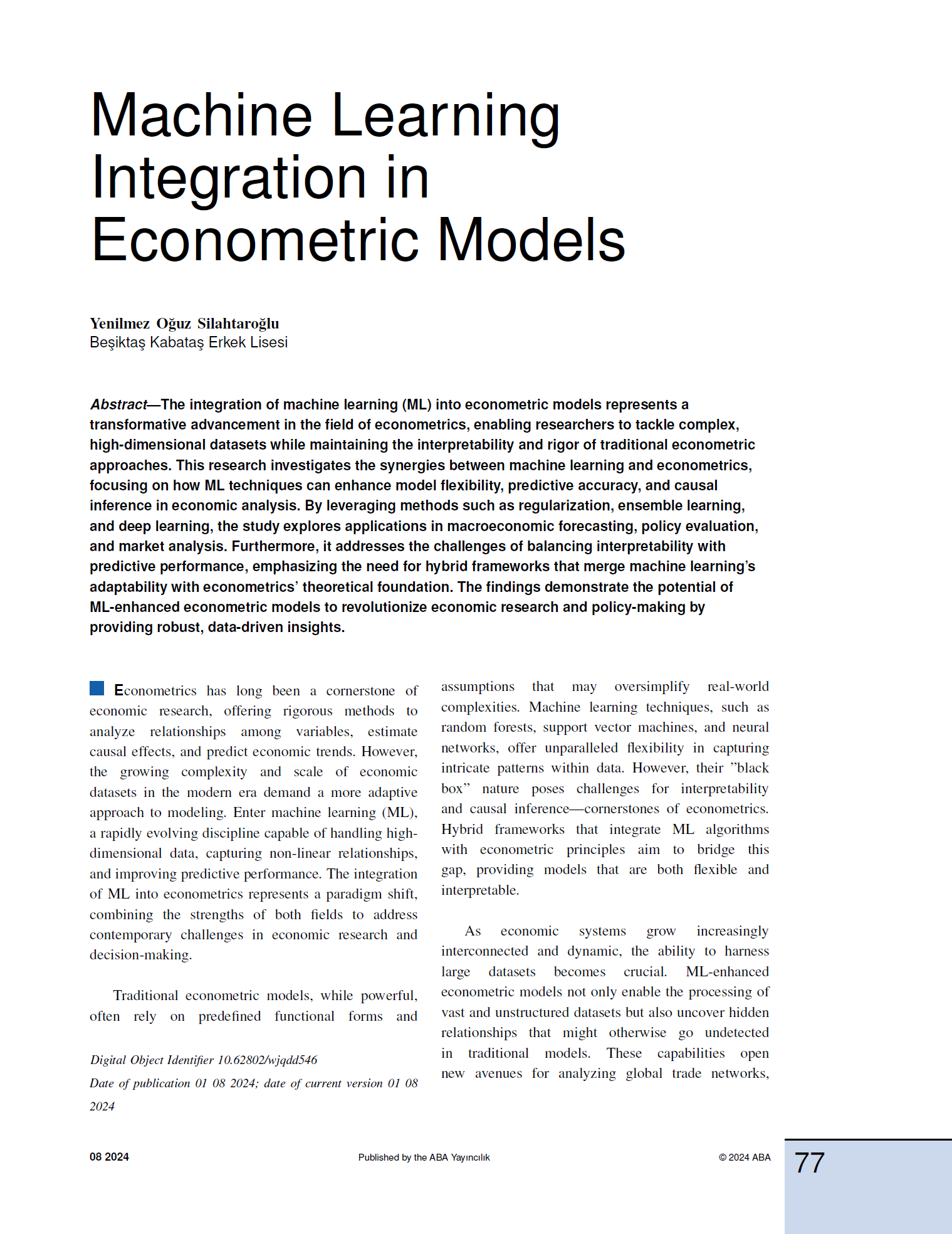