A Predictive Framework for Real-Time Health Monitoring via Wearable Biosensors
DOI:
https://doi.org/10.62802/jpqemk08Keywords:
Wearable biosensors, real-time health monitoring, predictive analytics, machine learning, preventive healthcare, biometric data, chronic disease management, personalized health, health anomaly detection, continuous trackingAbstract
Wearable biosensors, coupled with predictive analytics, are transforming real-time health monitoring by providing continuous, personalized insights into physiological metrics. This framework leverages wearable technology and advanced machine learning algorithms to process biometric data, enabling early detection of health anomalies and proactive intervention. Through the integration of biosensors monitoring parameters such as heart rate, blood pressure, glucose levels, and respiratory rate, the predictive framework can identify patterns indicative of potential health risks. Machine learning algorithms analyze real-time data streams, facilitating precise predictions and personalized feedback to users and healthcare providers. This approach not only enhances patient engagement and preventive care but also supports the management of chronic conditions by allowing continuous tracking outside clinical settings. Despite its potential, challenges such as data privacy, battery life limitations, and the accuracy of sensor data must be addressed. This research explores the design, functionality, and implications of a predictive health monitoring framework, examining the role of wearable biosensors in enabling proactive healthcare solutions.
References
Bansal, S., Saraswat, M., & Saraswat, B. K. Advancement in wearable biosensor for real time health monitoring. In Sustainability in Digital Transformation Era: Driving Innovative & Growth (pp. 97-103). CRC Press.
Gao, L., Wang, C., & Wu, G. (2024). Wearable Biosensor Smart Glasses Based on Augmented Reality and Eye Tracking. Sensors, 24(20), 6740.
Khan, A. O. R., Islam, S. M., Sarkar, A., Islam, T., Paul, R., & Bari, M. S. Real-Time Predictive Health Monitoring Using AI-Driven Wearable Sensors: Enhancing Early Detection and Personalized Interventions in Chronic Disease Management.
Kumar, T. S., Kumar, G. A., & Shaik, A. (2024). Remote Patient Health Monitoring Using Iot And Artificial Intelligence. Nanotechnology Perceptions, 79-92.
Priyadharshini, S. I., Irene, D. S., Beulah, J. R., & Ponnuviji, N. P. (2025). Enhancing real-time health monitoring with hybrid recurrent long short-term tyrannosaurus search for menstrual cups. Biomedical Signal Processing and Control, 100, 107065.
Raymond, D. A., Kumar, P., & Goureshettiwar, P. (2024, August). Intergration of Wearable Biosensors and Data Analytics for Remote Health Monitoring. In 2024 Second International Conference on Intelligent Cyber Physical Systems and Internet of Things (ICoICI) (pp. 707-713). IEEE.
Reddy, G. R., Humera, D., Reddy, M. S., Painam, R. K., & Kumar, K. D. Biosensor Applications in Embedded Wearable Devices. In Handbook of Artificial Intelligence and Wearables (pp. 316-325). CRC Press.
Riaz, M. S., Shaukat, M., Saeed, T., Ijaz, A., Qureshi, H. N., Posokhova, I., ... & Imran, A. (2024). iPREDICT: AI enabled proactive pandemic prediction using biosensing wearable devices. Informatics in Medicine Unlocked, 46, 101478.
Suryawanshi, N. S. Predicting Mental Health Outcomes Using Wearable Device Data and Machine Learning.
Theyagarajan, K., & Kim, Y. J. (2024). Metal Organic Frameworks Based Wearable and Point-of-Care Electrochemical Sensors for Healthcare Monitoring. Biosensors, 14(10), 492.
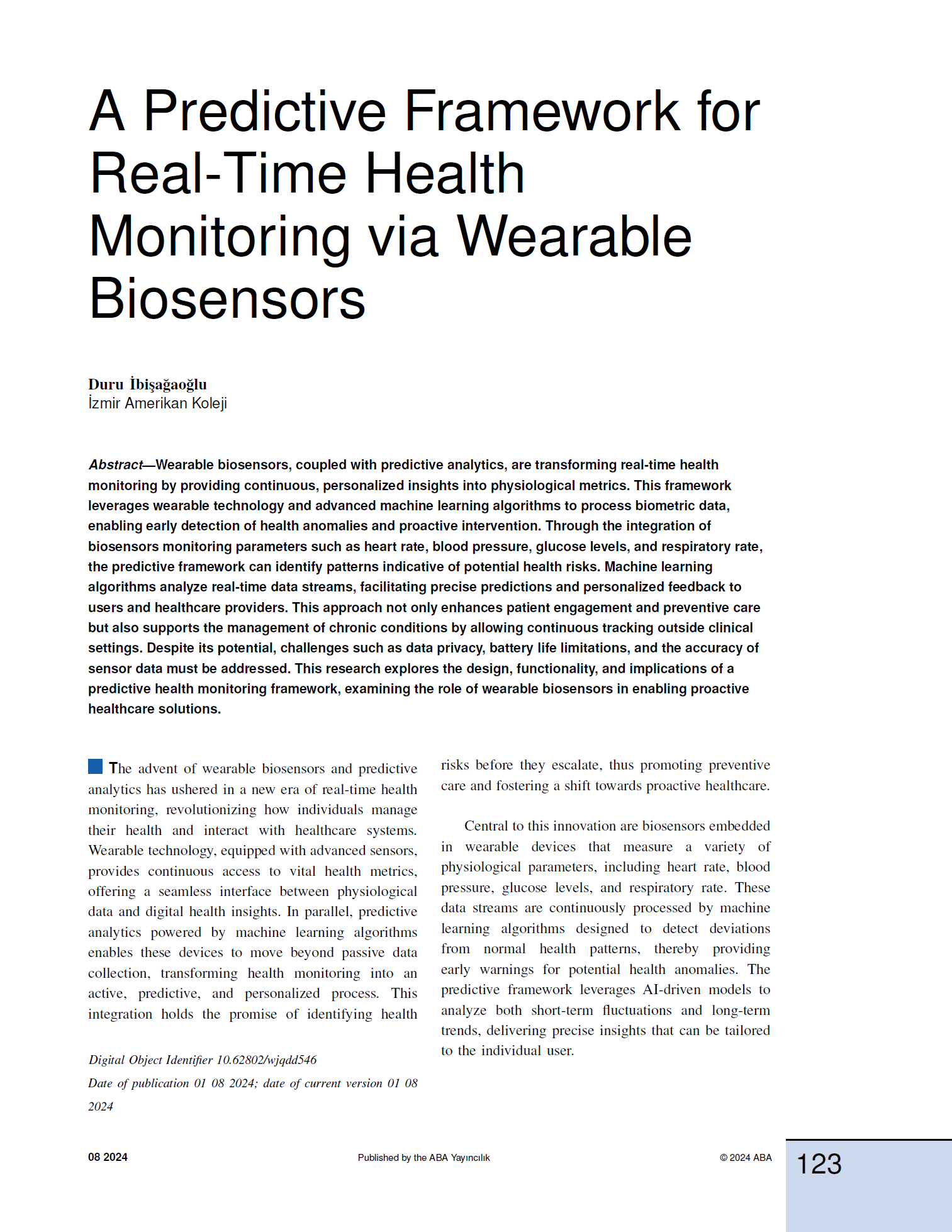