Uncovering Disease Mechanisms through AI and Visual Computing: A Multi-Scale Approach to Biological Data Interpretation
DOI:
https://doi.org/10.62802/419m8z78Keywords:
Artificial Intelligence in Healthcare, Visual Computing in Biology, Multi-Scale Data Analysis, Hierarchical Neural Networks, Biological Data Interpretation, Molecular to Organ-Level Analysis, Disease-Related Pattern RecognitionAbstract
This research focuses on uncovering complex disease mechanisms by employing artificial intelligence (AI) and visual computing to interpret multi-scale biological data. By analyzing data from molecular to organ-level systems, this study aims to bridge the gap between cellular insights and clinical applications, offering new perspectives on diseases such as cancer, neurodegenerative disorders, and cardiovascular diseases. Through a comprehensive approach to data integration, the project compiles omics and imaging data to enable holistic analysis across biological scales. The use of hierarchical neural networks, graph neural networks (GNNs), and convolutional neural networks (CNNs) allows for multi-dimensional modeling and the extraction of disease-related patterns. The findings are validated with clinical data to support actionable insights, laying the groundwork for personalized disease models and precision therapeutic strategies. Expected outcomes include breakthroughs in disease understanding, advancements in precision medicine, and the establishment of a robust AI framework that will contribute to future biomedical applications. This multidisciplinary approach promises to transform diagnostics, prognosis, and treatment, facilitating a deeper understanding of disease mechanisms and their translation into clinical practice. The integration of multi-scale data enables a nuanced view of disease, capturing interactions that may be obscured in single-level analyses. By employing advanced visual computing techniques, the project highlights structural abnormalities within tissue samples, linking cellular changes to systemic disease pathways. This approach not only aids in identifying novel biomarkers but also supports the development of individualized treatment models that predict patient-specific responses. With validation from clinical datasets, the AI framework is poised to enhance clinical decision-making, offering insights tailored to the unique biological profiles of patients. The project’s multi-scale methodology serves as a foundation for future biomedical research, setting a new standard for AI-driven disease analysis.
References
al., G. (2023). Integrative analysis of multi-omics data with deep learning: challenges and opportunities in bioinformatics.. tjjpt, 44(3), 1384-1392. https://doi.org/10.52783/tjjpt.v44.i3.488
Gao, Y., Sharma, T., & Cui, Y. (2023). Addressing the challenge of biomedical data inequality: an artificial intelligence perspective. Annual Review of Biomedical Data Science, 6(1), 153-171. https://doi.org/10.1146/annurev-biodatasci-020722-020704
Liu, N., Feng, Q., & Hu, X. (2022). Interpretability in graph neural networks., 121-147. https://doi.org/10.1007/978-981-16-6054-2_7
Wu, Z., Pan, S., Chen, F., Long, G., Zhang, C., & Yu, P. (2021). A comprehensive survey on graph neural networks. Ieee Transactions on Neural Networks and Learning Systems, 32(1), 4-24. https://doi.org/10.1109/tnnls.2020.2978386
Zhu, J. (2024). Decoding cell identity with multi-scale explainable deep learning.. https://doi.org/10.1101/2024.02.05.578922
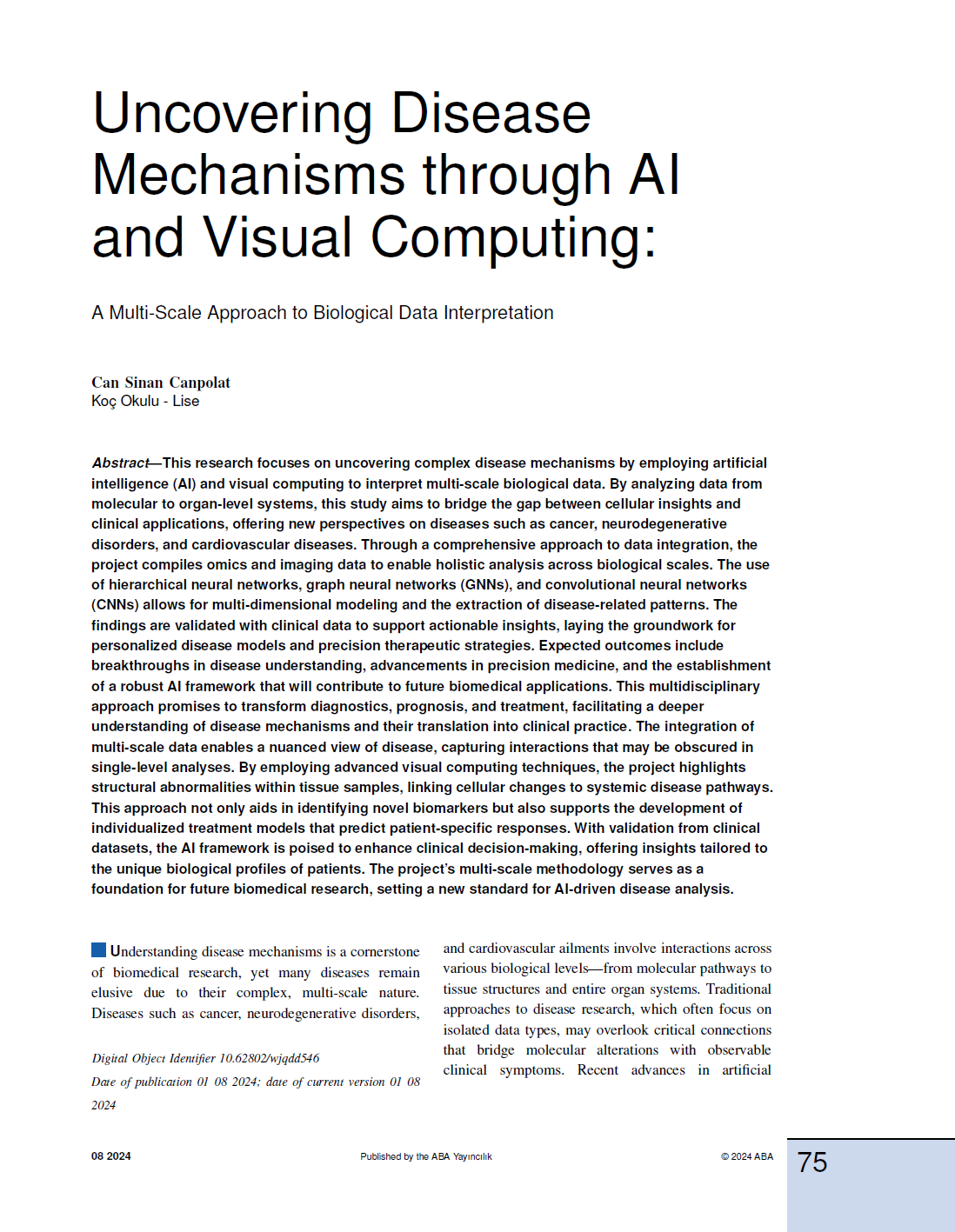